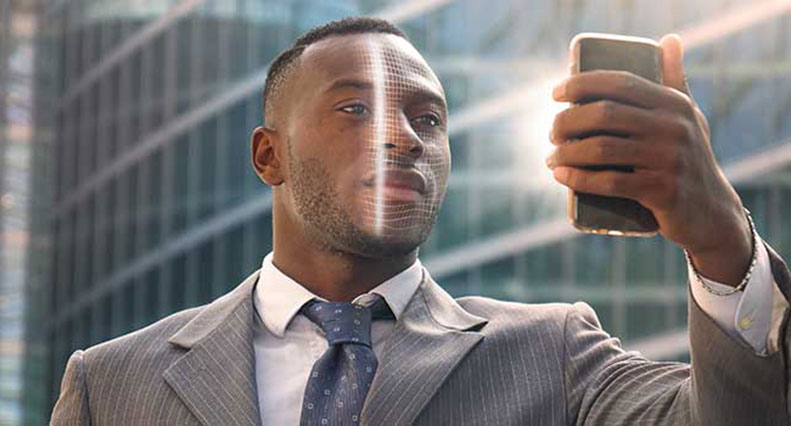
Face Recognition Technology: the Function and Relevance
The relevance of face recognition technology
Face recognition technology in one form or another has been developing for a long time. However, over the past ten years or so, a significant leap has taken place in the field of development and training of neural networks. This direction is already one of the most relevant and promising, along with the development of information transfer technologies, various cloud services, and meaningful analysis of large amounts of data.
Let’s try to understand what face recognition systems can do today in terms of video and image analysis and where the limit is.
Technology development
Both architecture and training methods of neural networks continue to actively develop and discover new applications in practice. For example, today the so-called recurrent neural networks are already used, which has significantly improved the quality of face recognition systems based on them.
For a long time, the logic of the work of neural networks provided for work with each frame individually and, accordingly, their separate analysis. As a result, the system often made erroneous decisions. The logic of the operation of recurrent networks makes it possible to fully take into account the context when the sequence of frames is considered as the total set and the result of processing previous frames affects the processing of subsequent frames. Thus, very high quality of recognition is achieved. And this, in turn, expands the possibilities of practical application.
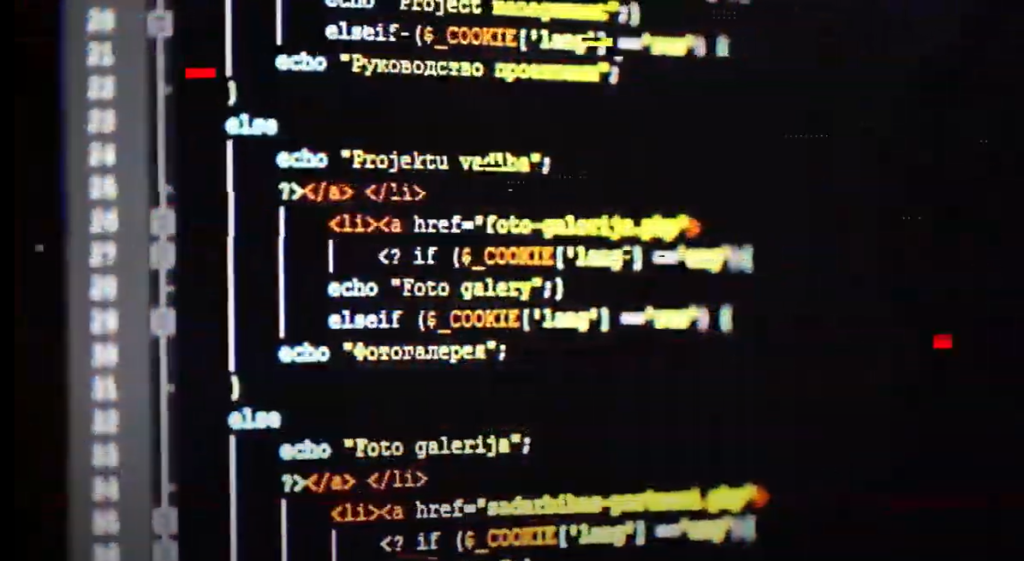
An approach to the use of computing resources is also being developed to utilize them as efficiently as possible. Face recognition systems use the power of both the CPU and the GPU. Good results can be achieved when one server can process significantly more video streams by redistributing various types of computational tasks between them, as well as optimizing the overall workflow of the recognition process.
How face recognition works
The top-level principle of the operation of face recognition systems can be described as follows. By a system, we mean not only the neural network itself but the entire set of components before and after it that are involved in the process. The encoded video stream from the camera, which is a sequence of frames on which can be “faces,” is received at the system input. The data goes to a so-called decoder, which decodes the video stream into separate frames for further processing by the detector. Next, the detector on each frame determines the presence of any objects in the frame that are similar to a face.
After that, scoring of the detected objects takes place, key points are determined that allow you to determine the angle of rotation of the face and its quality. Based on this, individuals are rated. Objects evaluated as individuals are thrown out completely. On the detected faces from a set of consecutive frames, a one-person tracklet is built. For example, when the same person passed from one end of the room to the other in the frame and entered the system immediately at 100 frames, duplication is eliminated and several best “faces” from the person tracklet are determined (the highest rating) that will be involved in recognition. Objects assigned to faces but not suitable for recognition (for example, the system understands that the face is in the frame but it is strongly rotated and will not be enough for high-quality recognition of the visible part of the face) get a low rating.
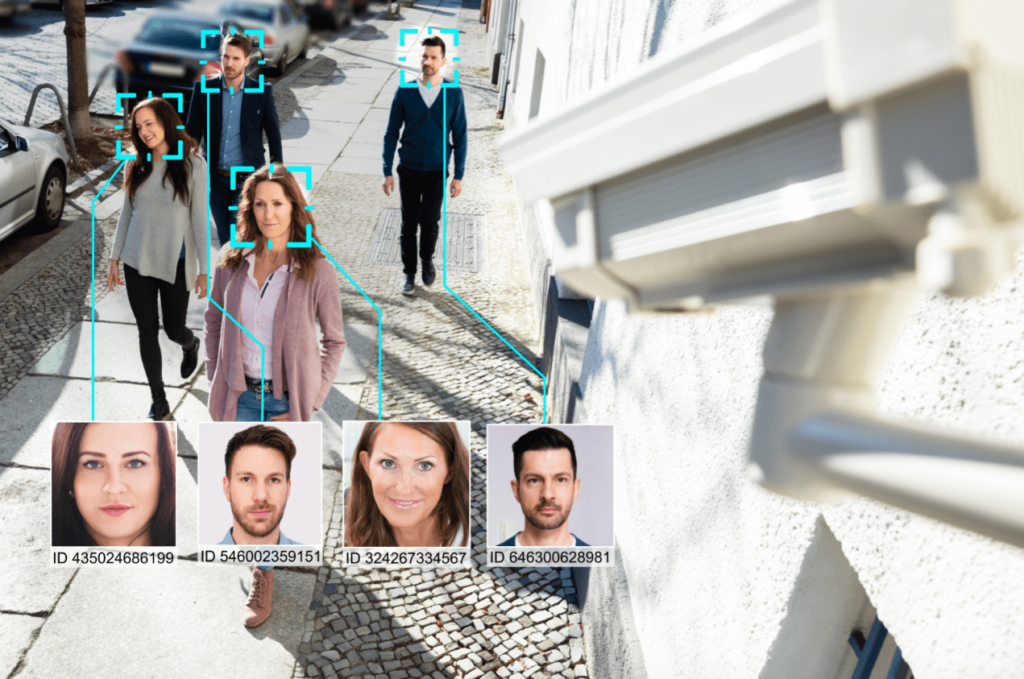
The selected “faces” from the tracklet are processed by the neural network, the so-called feature vector is determined from them – a unique set of features extracted by the neural network that is characteristic only of this person, which essentially forms a unique face code. Face recognition has occurred.
Further recognized faces through the same unique face code are compared with the database of persons in the system. And, if the difference does not exceed the specified threshold value, the system associates the person recognized in the frame with the person from the database. If it exceeds, a new person is formed. A personification of the person has occurred.
Thus, the neural network is only part of the face recognition system. Most often, there are several networks in the system and each is important to achieve a quality result. Let’s say a good feature vector extraction network and a bad detector network will not give a good result. The quality-trained networks allow you to get a high level of face recognition.
Is it possible to “beat” the system?
The answer to this question is highly dependent on the context of the task. There are two extreme cases. If the face is completely or almost completely covered, then the system does not recognize it (does not even detect it), which is logical. If the face is completely open (and still has a high rating), then the system will accurately recognize it correctly. Between them, there are many different facial states in the frame.
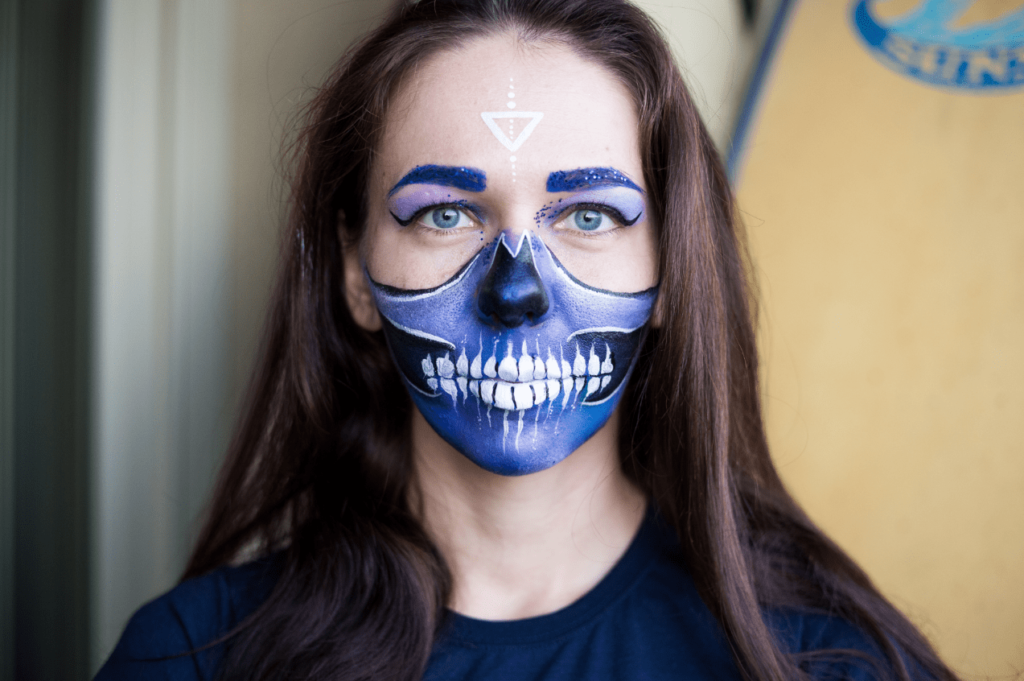
As noted above, the neural network extracts a whole set of values that form the vector of features of one person. There may be several tens or even hundreds. If part of the face is not visible well (for example, it is covered with something or an unusual pattern is applied to the face designed to beat the network), then the analysis will still be made on the remaining parts. Although, the likelihood of error increases but most often uncritical.
For example, if you take some ready-made facial recognition system with a specific set of networks (each of which performs its function) and logic in the work, then the image of a person with artifacts applied to it can lead to incorrect determination of key points. The output system will give a bad result because at one of the stages there was a failure. However, if the problem stage is “disabled” in the same recognition system, then most likely it will correctly recognize the same face with artifacts. Also, there are many such examples. Therefore, there is probably no universal way to beat face recognition systems. To beat a specific system, it is necessary to understand in detail its logic of work, and this greatly complicates the task.
What technology is good for business?
Face recognition systems can help businesses automate some of the processes in companies that are now run by living people. Thus, reducing the participation of people in them or (in the future) generally replacing people in such areas. For example, such systems can be useful in store safety by conducting an automatic analysis of incoming visitors and comparing them with the “blacklist” for a more rapid response of personnel. Or in marketing issues, when the algorithm allows you to recognize the emotions of the client by analyzing gestures, facial expressions, eyes, etc. This will allow staff to better respond to targeted situations and make good proposals. It can conduct a retrospective analysis of the number of unique and loyal visitors to restaurants or cafes and record the working hours of their employees.
Practical implementations in industries
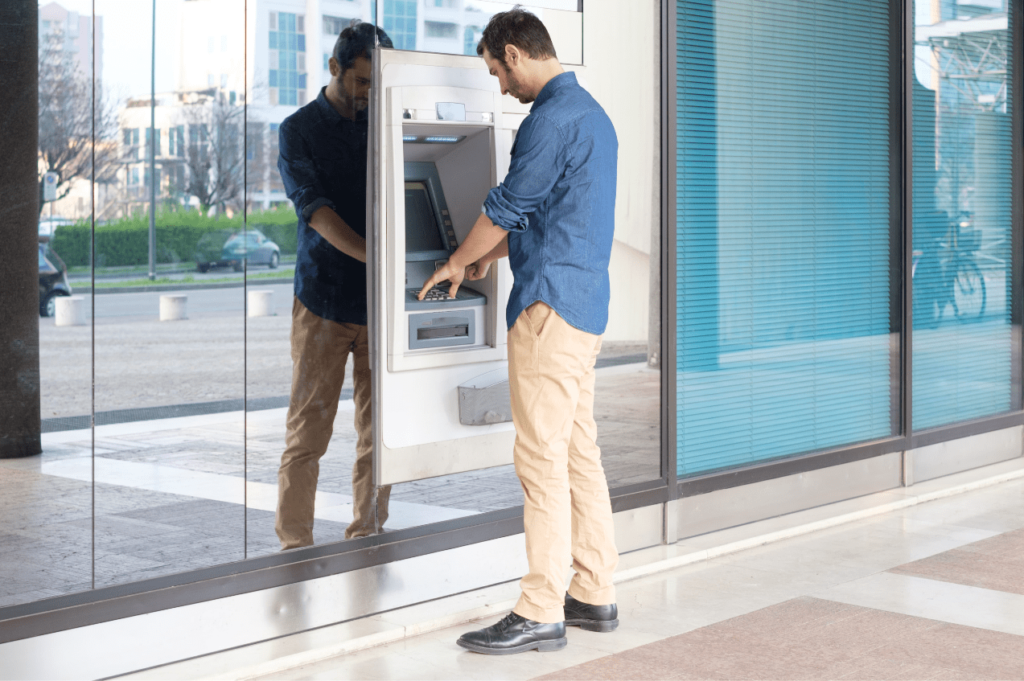
The areas of application for face recognition solutions are steadily expanding. Today, among them are the banking sector, transport, retail, and even medicine.
For example, by installing such a solution at an ATM, on the one hand, it is possible to simplify interaction with a client, and on the other hand, even increase its effectiveness. The client of the bank can be identified as soon as he approached the ATM. Moreover, by this moment the bank already knows a lot about the client: about his balances, preferences, connected services, etc. All this, together with secondary factors, such as the place, time, or day of the month, can offer the client something much more suitable. Such solutions have not yet found widespread application but they are already undergoing pilot testing.
A security camera with facial recognition is already installed on the metro tripod turnstiles in Moscow. Of course, initially, the main task was to ensure transport safety and help in finding offenders (when the system compares the faces of passengers with a database). However, pilot tests are currently being conducted to pay for the fares using this technology. We must say that the cameras on the subway turnstiles operate in conditions close to a perfect for recognition. However, even under such conditions, the probability of errors is still quite high (for example, today’s systems have significant limitations on the qualitative recognition of faces in a large crowd – this is a separate complicating condition), and the price for the mistake in cases of payment is high. Therefore, such technology will appear but a little later.
No one can any longer be surprised by modern intercoms that are controlled through the application on a smartphone from anywhere in the world (you only need Internet access). Also, the most advanced companies are already testing intercom solutions with face recognition allowing them to open the door using the face as soon as a person approaches it. Such models are not yet ready for mass use (in particular, in addition to the technology itself, it is also necessary to select the optimal scenario for the user – to approach the door to open it or to press any button) but there are already pilot zones.
Conclusion
Over the past few years, face recognition technologies have made significant advances both in terms of quality and in terms of the resources used (which means that they are becoming cheaper). Nevertheless, they are still quite far from the level of a living person. Therefore, today such solutions are advisable in business only for solving some narrow issues but they already exist today. However, the general trend nevertheless suggests that over time, solutions based on such technologies will increasingly become an assistant to people in a variety of tasks. First, it will concern simple and routine tasks, so that people can focus their efforts on more complex or creative ones.